Modern industrial production is advancing vigorously towards large-scale, high-speed, and intelligent direction. As productivity and intelligence gradually improve, major manufacturers are increasingly demanding safety and reliability of their mechanical equipment, especially for some large rotating machinery such as electric motors, fan units, hydraulic pumping stations, and so on. They have become key equipment in modern large-scale production facilities, requiring long-term continuous operation. Once a failure occurs, it may cause significant economic losses and even major safety accidents.
In fact, major equipment failures in the industry do not occur suddenly, but rather through a gradual deterioration process during which equipment can still operate with faults. However, in traditional equipment maintenance work, it is impossible to predict or forecast when failures or deterioration will occur. With the upgrade and transformation of intelligentization entering traditional industries, industrial equipment maintenance will inevitably move from “prevention” to “prediction”. The health status and operating conditions of equipment will be monitored in real-time anytime and anywhere, making maintenance more evidence-based and logical. Of course, this also puts higher demands on modern mechanical equipment solutions.
Paifang Technology, established in 2018, is an enterprise focused on providing intelligent solutions for industrial equipment. It has developed a complete set of equipment intelligent solutions for equipment manufacturing enterprises and factories, including an intelligent IoT terminal based on edge computing, artificial intelligence algorithm engine, and intelligent industrial equipment management cloud platform. This can effectively solve the needs of users for equipment data collection, intelligent analysis, efficient management, and precise decision-making, providing industrial users with full-stack equipment intelligent services. It has provided services to more than 100 well-known domestic and foreign enterprises in equipment manufacturing, coal mining, petroleum and petrochemicals, steel and metallurgy, and many other industries.
The core team of Paifang Technology comes from Tsinghua University and has world-leading artificial intelligence chip and algorithm technology. It has obtained nearly 40 independent research and development intellectual property rights and has won many titles, including national high-tech enterprises, Tianjin gazelle enterprises, and Tianjin strategic emerging industry leading enterprises.
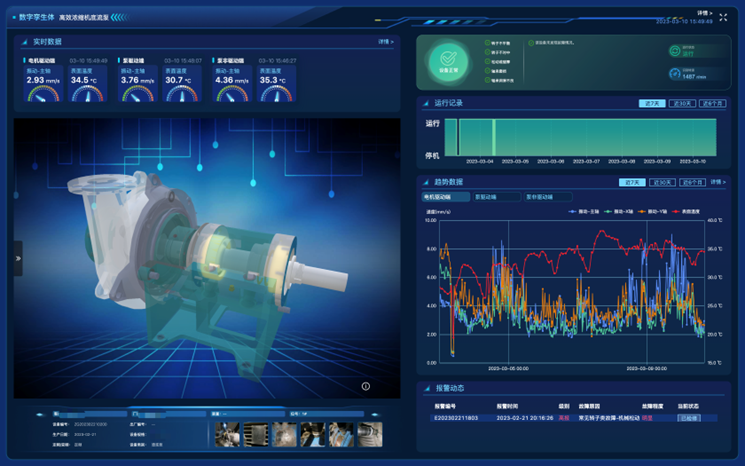
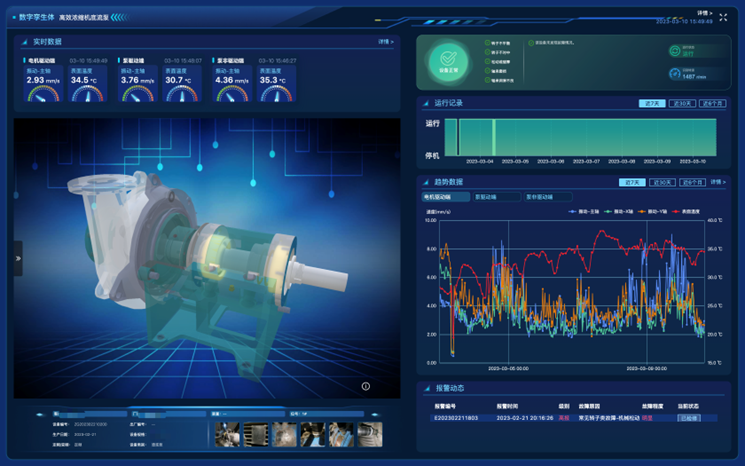
Business and Application Requirements
The predictive maintenance of rotating machinery mainly relies on deploying one or more measuring points on the equipment to collect data such as vibration and temperature. Through real-time analysis and judgment of the data, abnormal equipment conditions can be predicted and equipment failures can be accurately located. Take pumps as an example. A pump unit consists of an electric motor and a pump, and depending on the bearing configuration and equipment structure, 3–4 wireless temperature and vibration integrated sensors need to be deployed. Since each wireless temperature and vibration integrated sensor can collect 3 channels of high-frequency vibration signals above 10kHz and 1 channel of temperature signal, to ensure service quality, the acquisition frequency of vibration signals often needs to be above 20kHz, which means that each vibration signal needs to collect more than 20,000 data points per second, forming a large data scale.


Architecture of Solution
The industrial equipment intelligentization solution of Paifang Technology is mainly divided into 4 layers. First, the hardware device data at the edge is transmitted to the data integration layer through gateways and other means to achieve the collection of relevant data.
The data is then transmitted to the data center, which provides PASS services such as version management, CI/CD, and quality correction, as well as metadata management. More importantly, the data center supports deep learning engines such as TensorFlow and PyTorch. We should also note the selected database at this layer, which is the core of data storage, and its performance and stability will directly affect the overall architecture. Previously, Paifang Technology used traditional relational databases for data storage and did not introduce time series databases.
Next is the data access and analysis service layer, which provides various real-time data query APIs and access services, as well as relevant algorithm libraries for analysis services.
Finally, the Paifang platform business that supports the upper layer provides functional modules for user demands, such as equipment management, fault prediction, spare parts management, and other application sets. In addition, relevant monitoring tools run through the entire architecture.
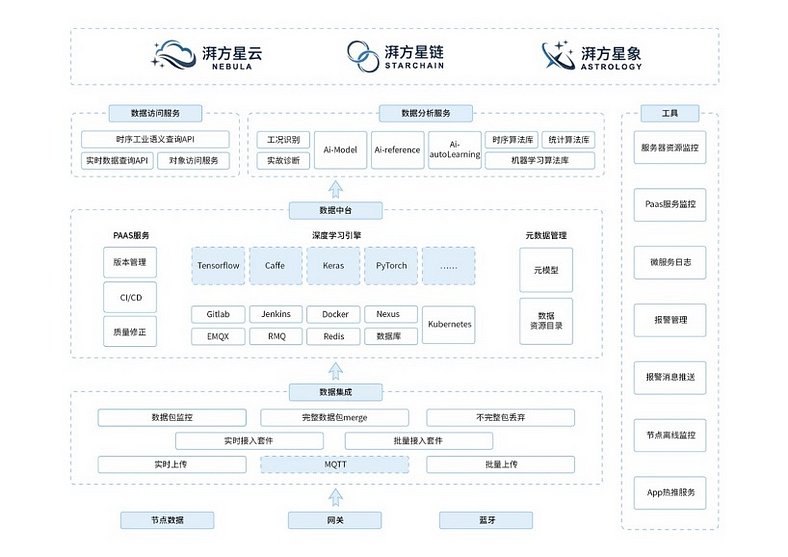
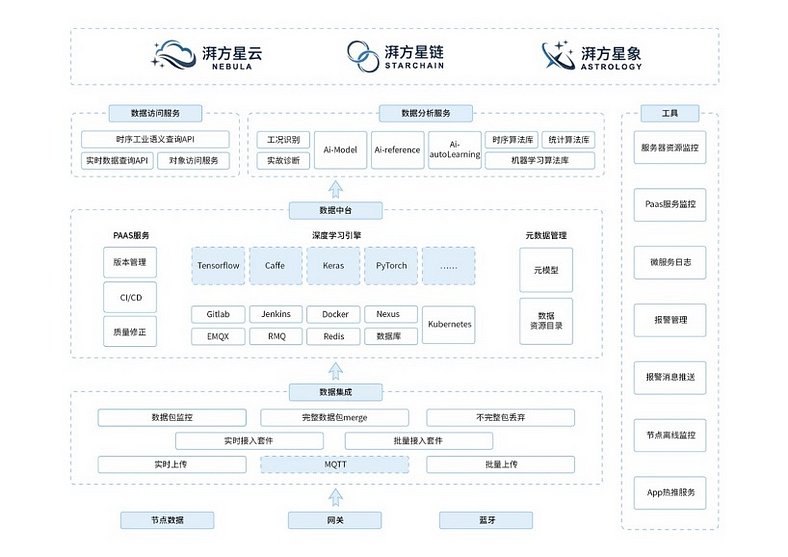
Challenges Faced by Traditional Architecture
Traditional architectures are facing challenges as the rapid development of industrial intelligence has led to a sharp increase in the number of intelligent devices connected to the cloud. One major challenge is the bottleneck of write-throughput capacity, as massive amounts of data cannot be efficiently written to traditional relational databases. For instance, in a typical production facility with 1,000 devices, each with 3,000 to 4,000 intelligent sensors that collect data every 30 minutes, there are approximately 150,000 writes per second, or ten thousand QPS. However, traditional relational databases such as MySQL and Oracle, which are constrained by storage structures, cannot efficiently store massive amounts of time-series data.
Another challenge is the high cost of storage, which prevents enterprises from economically preserving historical data for a longer period. To achieve more precise predictive maintenance and enable large-scale AI applications in the future, most enterprises hope to preserve longer historical data. This is especially true for high-end mechanical equipment with a net value of millions of dollars or critical equipment located in key production processes, with a lifespan of 10–20 years and high annual operating costs. However, due to the high storage cost and access cost of common disk arrays, users are often unable to save data for more than five years. Additionally, database compression ratios are not high, and storage costs are exorbitant. With the passage of time, these costs will continue to rise, causing users to lose large amounts of effective device data.
Using CnosDB to Solve the Problem
To address these challenges, Paifang Technology uses a new generation of time-series databases, such as CnosDB, to improve storage throughput and reduce storage costs. CnosDB is a time-series database that is very suitable for IoT scenarios, with robust write-throughput and efficient compression ratios. Compared with traditional relational databases, CnosDB can support larger-scale measuring points and write-throughput requirements of up to a million QPS on ordinary servers, making it possible for Paifang Technology to efficiently address platform scalability and data write-in for high-end precision devices.
In terms of table design, CnosDB is different from traditional databases, with each data upload requiring a record to be kept as metadata, and the device’s data indicators stored separately in another table.




With the new architecture design, CnosDB’s data compression ratio reaches 60x, which reduces the storage space occupied by data and therefore lowers storage costs. Longer and larger data retention periods will also enable us to explore deeper data values. Taking the storage of 55T data over 3 years and 50 billion records as an example, after using CnosDB, the storage space occupied by the data under double replicas is reduced to about 6.2T. After adopting the CnosDB solution, Paifang Technology’s storage costs have decreased by 90%. Advanced predictive maintenance can also be achieved due to the high-quality data, which can ultimately delay equipment aging.
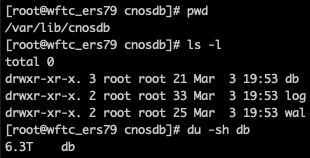
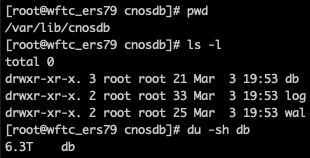
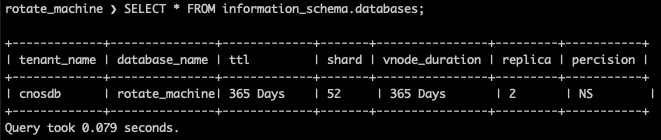
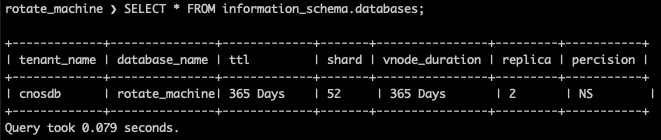
In addition, in terms of query performance, most queries have also seen a speed increase of around 20 times compared to the original architecture.


Future of Work
The application of CnosDB has made it possible for industries to collect and retain data with higher accuracy and for longer periods of time. The development of cloud IoT environments and digital twin technology has given us the opportunity to view a virtual representation of a device’s different operating parameters in near real-time. A digital twin is a visual replica of a physical device, and by combining data such as vibration, power, and temperature with information about the device, we can create a visual “twin” for each device. We can establish operational standards to indicate normal operation or parameters that exceed expected thresholds, and monitor the virtual replica of the device anytime, anywhere, eliminating the inconvenience or unnecessary travel to factory workshops.
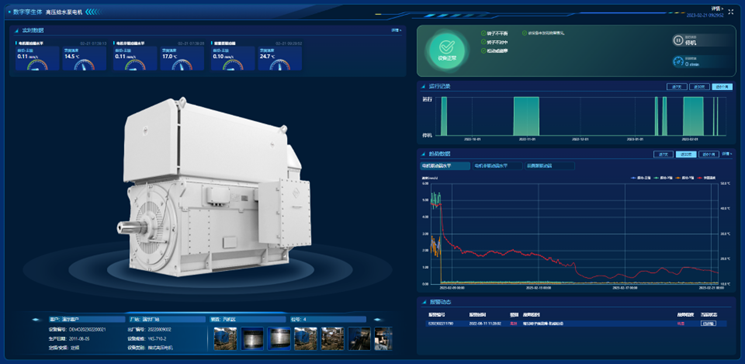
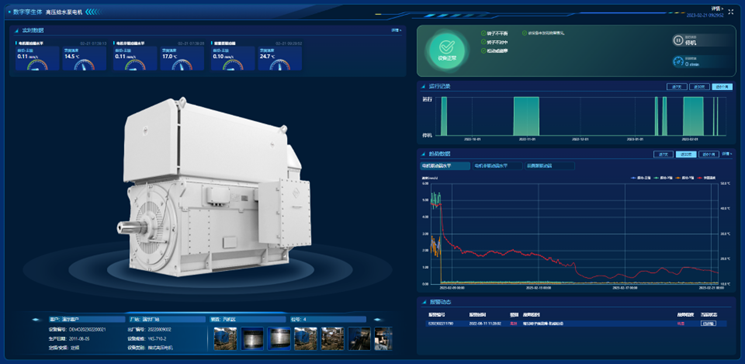
In addition, the development of artificial intelligence is also worth looking forward to. From simple charting functions to AI analytics, we need to find time-series tools for trend analysis. For more expensive industrial equipment, the use of AI analysis may also help us quickly identify anomalies, thereby providing customers with better predictive maintenance services.
Author Introduction
Wu Tongda is the CEO of Paifang Technology. He graduated from the Department of Electronic Engineering at Tsinghua University in 2010 with a bachelor’s degree and completed his doctoral studies in 2017. With 6 years of experience in low-power circuit system design and 4 years of entrepreneurial practice, he was named one of the “30 under 30” new business leaders by Entrepreneurship State in 2021 and a Hu Run U30 Chinese entrepreneurial pioneer in 2022. As the first author, he has published 2 papers indexed by SCI and 2 indexed by EI. He participated in the writing of a national standard, and has been granted 8 patents, including 4 invention patents. He is also the director of the Institute of Intelligent Chips and Industrial Internet of Things at Tsinghua University Tianjin Electronic Information Research Institute, and was the co-founder of Rational Design, project director of Tsinghua Future Communication student team, and chairman of the 4th Asian Intelligent Sensor System Seminar.
Product-related documents:
- CnosDB Quick Start Guide: https://docs.cnosdb.com
- CnosDB Official Website: https://www.cnosdb.com
- CnosDB GitHub Repository: https://github.com/cnosdb/cnosdb